The Power of Monte Carlo Simulations in Higher Education
In an era of increasing uncertainty, managing financial risk has become critical for Higher Education (HE) institutions, especially in the UK where shifting demographics, funding changes, and external shocks such as Brexit have strained traditional forecasting models. The financial sustainability of universities and colleges depends on robust risk management practices that can adapt to these uncertainties. One powerful tool to achieve this is Monte Carlo analysis, a method that enables institutions to forecast a range of possible outcomes, quantify risks, and make informed financial decisions.
What is Monte Carlo Analysis and How Does it Work?
At its core, Monte Carlo analysis is a computational technique that uses random sampling to simulate a variety of outcomes in situations where multiple uncertainties exist. Instead of relying on static point estimates, this method models outcomes using probability distributions, which are a way of expressing all the possible outcomes and their likelihoods.
A probability distribution reflects the range of possible values for a variable and the likelihood of each value occurring. These distributions are fundamental to Monte Carlo simulations, as they quantify both the uncertainty and the probability of different outcomes. In financial forecasting, common distributions include Gaussian (normal distribution), which assumes most outcomes cluster around the average, and Beta distribution, which allows for more customization in terms of skewness and concentration of probabilities. Understanding these distributions allows HE institutions to reflect real-world uncertainties more accurately than simple, linear models.
How Can Monte Carlo Analysis Be Used in Financial Forecasting?
Monte Carlo analysis can enhance financial models by replacing rigid assumptions with dynamic, probabilistic inputs.
Assumptions with Distributions: Instead of assuming that a particular future outcome, such as government grants, will be a single fixed value, Monte Carlo simulations allow institutions to model this assumption with a distribution—reflecting the inherent uncertainty. For example, a Beta distribution could be used to model grant income, where funding tends to fall within a predictable range but with a notable risk of low or high outliers. Similarly, for an expense line such as staff expense, institutions might apply a Gaussian distribution to reflect the fluctuations in employer pension contributions and wage inflation.

Aggregated Outputs: What makes Monte Carlo analysis particularly powerful is how these individual distributions are aggregated. When multiple assumptions—each with their own uncertainties—are combined, the simulation produces a nuanced, aggregated view of potential outcomes. This could be used to forecast overall cash flow, factoring in the variability in revenue, operating expenses, and other financial commitments. The result is not a single projection, but a spectrum of possible outcomes, with probabilities attached to each, allowing for more comprehensive risk assessment.
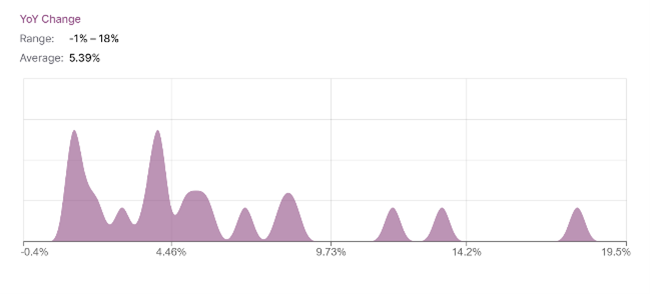
What Insights Can Monte Carlo Analysis Provide?
By incorporating probability distributions into financial forecasts, Monte Carlo analysis enables institutions to:
Understand Variability in Outcomes: Instead of relying on deterministic forecasts, HE institutions can see the range of potential financial outcomes over different time horizons. This enables leaders to anticipate how variations in student enrolment, tuition fees, or grant income could impact their financial position.
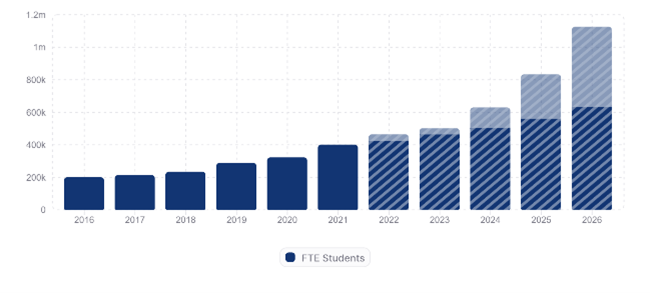
Quantify Risk: Monte Carlo provides a clear view of the risks tied to financial assumptions. For example, if enrolment numbers are uncertain due to demographic shifts, the model can show the likelihood of different enrolment levels and their subsequent impact on tuition revenue. Similarly, understanding potential fluctuations in operational expenses can help forecast financial health under both best- and worst-case scenarios.
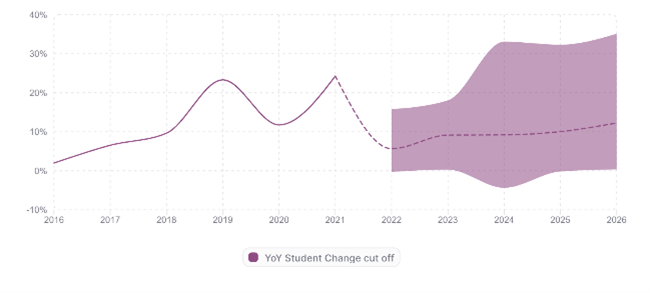
How Can Monte Carlo Analysis Improve Decision Making?
Monte Carlo simulations not only clarify potential outcomes, but also equip decision-makers with the tools to plan for sustainability.
For example, HE finance directors can model how much cash reserve is required to maintain operations under different scenarios. By understanding the probability of various levels of grant income or changes in student enrolment, institutions can more effectively plan how much to set aside to cover potential shortfalls. These insights can guide decisions around long-term investments, hiring, capital projects, and even debt management—ensuring that institutions are better prepared for both expected and unexpected events.
Moreover, this approach helps assess how resilient an institution’s finances are against external risks. Whether it's shifts in government policy, economic downturns, or changes in student demand, Monte Carlo analysis offers a way to not just see risks in isolation, but to understand how multiple risks could interact and affect financial sustainability. With a clearer view of the risks, decision-makers can take more confident steps toward mitigating them.
Conclusion
Monte Carlo analysis transforms financial forecasting by adding a layer of sophistication that standard models cannot achieve. For Higher Education institutions in the UK, this quantitative approach to risk management is particularly valuable, offering the ability to forecast a range of potential outcomes while taking into account the inherent uncertainties of the sector. In an increasingly complex financial environment, HE institutions that adopt Monte Carlo simulations can not only manage risks better, but also position themselves for long-term sustainability.
For HE finance directors, the message is clear: embracing probabilistic methods like Monte Carlo is not just a technical enhancement—it is a strategic necessity.
コメント